Aluminum is the most cost-efficient base metal in terms of processing for re-use. The key is to use this concept in terms of re-melting—that is, melt specifically the scrap that is the desired alloy of production—and save money on fuel and reduce carbon footprint loads as a result.
So far, the sorting technologies used in Aluminum recycling are generally based on physical properties, just like density characterization by XRT (X-Ray Transmission). It is known that these physical characterization techniques are not able to recognize most elemental alloying differences that make up the wide variety of characteristic inter-, and intra-alloying classes.
In terms of chemistry-based sorting, XRF (X-Ray Fluorescence) as a sensor has significant limitations for most light fraction alloys such Cast and Wrought Aluminums, Magnesium- and Titanium-based alloys.
LIBS (Laser Induced Breakdown Spectroscopy) has been developed over many years of R&D to yield key advantages in light metal sorting. It has proven the ability to sort fine differences of alloying elements of interest such as Fe, Mg, Mn, Cu, Zn, etc. Differentiation of these in the in-feed scrap metal loads has the utmost of importance when dedicated recycling processors need short furnace turns. With the application of the LIBS, it’s possible to very selectively create a variety of the wide range of alloy classes from scrap.
Thanks to its unrivaled high quality and performance, Austin AI’s patented LIBS sensor-based sorting system has the most satisfied clientele and return customers. As one of our many examples of success, a specific sample test summary is given below.
Method
Study the performance of Austin AI’s LIBS sensor sorter system when testing samples with different coating layers on.
Sample description
Groups of unknown Aluminum alloy samples from different scrap sources were investigated with special focus on their variant coatings.
Instrument details
Austin AI at its Austin office has a 1-channel LIBS sensor sorter system for test and demonstration purposes. This demo LIBS system is a modular set up, including feeder, LIBS module, chute and the proprietary algorithm.
The demonstration unit is a single channel demo system that has exactly the same design and components as any production unit. The only difference is that all production units are built with the capacity to hold up to 6 sensors in one sensor-sorter configuration.
Test Procedure
First we labeled each separate piece for easy identification during our sorting tests. After some preliminary testing on the whole, mixed lot of material we soon discovered that there were at least 4 separate sample surfaces possible for the LIBS sensor to see. These are:
- Clean, relatively bare metal.
- Thinly coated material—paint and polymer on one side.
- Thinly coated material—paint and polymer on both sides.
- Heavily coated material, with the coating believed to be a powder coating.
- Steel samples, 2 total. We removed these from subsequent testing.
We have thoroughly tested the samples. First, we realized that Fe was not the proper qualifier for sorting the high alloying material from the low alloying material. Tests showed very little differences in the Fe signal.
Taking a hint from one of our former experiences, when sorting auto wheel scrap via LIBS, we decided to look at the Cu content. Here there was promising data on the few samples tested. So we decided to use that key EOI as our indicator.
After several test runs, we inspected the samples to see what impact the coatings had on LIBS analysis.
Clean Material—LIBS etches down to bare metal Corroded Material—LIBS etches down to bare metal Painted Material—LIBS etch down to bare metal Painted Material—LIBS etches down to bare metal Polymer Material—LIBS etches down to bare metal Powder Coated Material—LIBS does not etch down to bare metal, unless the bare metal is exposed
The observation is that the only problem material is the thicker, powder coated material where no clean surface is seen. Based on the small population of these among about 240 samples tested, we have concluded that when these do appear, they will not seriously affected the final chemistry of the pass material (that <0.15% Fe).
Testing results
Test 1. Run all “high Fe” material several times to get an average performance report. Data as follows:
- Samples: ~140
- Eject: 118 or 84%
- Blown but not diverted: 11
- Passed as “low Fe”: 22 or 16%
Test 2. Run all “low Fe” material several times to get an average performance report. Data as follows:
- Samples: ~100
- Eject: 16 or 16%
- Blown but not diverted: 2
- Passed as “low Fe”: 85 or 85%
Test 3. Run all material several times to get an average performance report. Data as follows:
- Samples: ~240 total; High = 140; Low = 100
- Eject:
- High Fe: 120, or 86%
- Low Fe: 16, or 16%
- Passed:
- High Fe: 20, or 14%
- Low Fe: 82, or 82%
Conclusions
The test results are very positive for using Cu as the criteria for effectively sorting designated high and low Fe cast alloy samples. Basically, as is, we recover the low Fe around 86% at 82% purity. If we correct this data by the documented diversion errors of about 10%, then we are well into the ~90% figure of merit for both parameters. This performance will ensure that these mixed scrap can be sorted into a low Fe group that will achieve low Fe melt specifications.
We removed the two steel samples from testing assuming this was a customer sampling error. However if this is not the case and these sample types do get included in the mix with the wheels, on occasion, then we can also open up the Fe channel and make sure we eliminate these along with the high Fe material.
Addendum
The customer supplied a new suite of samples to augment the testing from above. These samples were all classified as cast Al material and identified simply as:
- <0.11% Fe (orange)
- <0.15% Fe (yellow)
- >0.24% Fe (none)
Given the small differences in Fe concentration, extensive research was done to determine the best methodological approach for maximum performance in both purity AND efficiency of extraction. Samples were touched with paint for easy visual identification during testing various sortation parameters. Coloring codes are given above.
The best method for proper sorting for all three classes was as follows:
- Run all supplied samples mixed together and in Program 1, extract (divert) the lowest Fe (<0.11%) content first.
- Re-run the remaining “pass” material under Program 2, extracting (diverting) the second lowest Fe. (<0.15%) second, with the “pass” fraction being the highest Fe concentration material.
Addendum Results
Program 1
- Run rate: ~700 kg/hr (>4 TPH on a 6 channel system)
- Efficiency of extraction (avg): 92%
- Purity of extracted material (avg): 96% <0.11% Fe
Program 2
- Run rate: ~700 kg/hr (>4 TPH on a 6 channel system)
- Efficiency of extraction (avg): 95%
- Purity of extracted material (avg): 95% <0.15% Fe
Addendum Conclusions
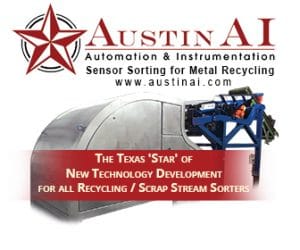
As previously reported in the initial testing results above, the Austin AI LIBS based sensor-sorter has proven capable of meeting the client’s demanding sortation requirements.
Please reach out to us for further details at ‘info@austinai.com’. Austin AI, Inc., 8862 Highway 290 West, Austin, Texas 78736 USA